Google DeepMind is now in a position to prepare tiny, off-the-shelf robots to sq. off on the soccer discipline. In a brand new paper printed right this moment in Science Robotics, researchers element their latest efforts to adapt a machine studying subset often known as deep reinforcement studying (deep RL) to show bipedal bots a simplified model of the game. The crew notes that whereas comparable experiments created extraordinarily agile quadrupedal robots (see: Boston Dynamics Spot) up to now, a lot much less work has been carried out for two-legged, humanoid machines. However new footage of the bots dribbling, defending, and capturing objectives exhibits off simply how good a coach deep reinforcement studying may very well be for humanoid machines.
Whereas finally meant for enormous duties like local weather forecasting and materials engineering, Google DeepMind may completely obliterate human opponents in video games like chess, go, and even Starcraft II. However all these strategic maneuvers don’t require complicated bodily motion and coordination. So whereas DeepMind can research simulated soccer movements, it hasn’t been in a position to translate to a bodily enjoying discipline—however that’s rapidly altering.
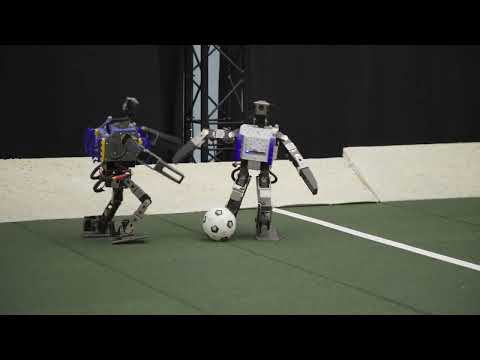
To make the miniature Messi’s, engineers first developed and skilled two deep RL talent units in pc simulations—the flexibility to stand up from the bottom and how you can rating objectives in opposition to an untrained opponent. From there, they nearly skilled their system to play a full one-on-one soccer matchup by combining these talent units, then randomly pairing them in opposition to partially skilled copies of themselves.
[Related: Google DeepMind’s AI forecasting is outperforming the ‘gold standard’ model.]
“Thus, within the second stage, the agent discovered to mix beforehand discovered expertise, refine them to the total soccer activity, and predict and anticipate the opponent’s habits,” researchers wrote of their paper introduction, later noting that, “Throughout play, the brokers transitioned between all of those behaviors fluidly.”
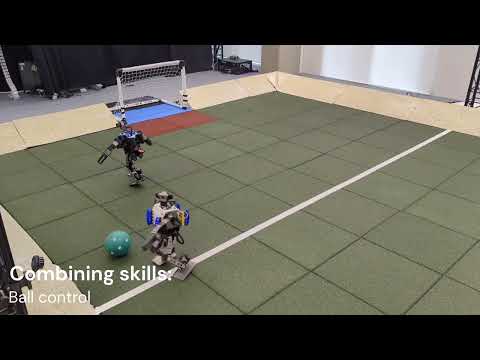
Due to the deep RL framework, DeepMind-powered brokers quickly discovered to enhance on current talents, together with how you can kick and shoot the soccer ball, block photographs, and even defend their very own purpose in opposition to an attacking opponent by utilizing its physique as a protect.
Throughout a sequence of one-on-one matches utilizing robots using the deep RL coaching, the 2 mechanical athletes walked, turned, kicked, and uprighted themselves quicker than if engineers merely equipped them a scripted baseline of expertise. These weren’t miniscule enhancements, both—in comparison with a non-adaptable scripted baseline, the robots walked 181 p.c quicker, turned 302 p.c quicker, kicked 34 p.c quicker, and took 63 p.c much less time to stand up after falling. What’s extra, the deep RL-trained robots additionally confirmed new, emergent behaviors like pivoting on their ft and spinning. Such actions can be extraordinarily difficult to pre-script in any other case.
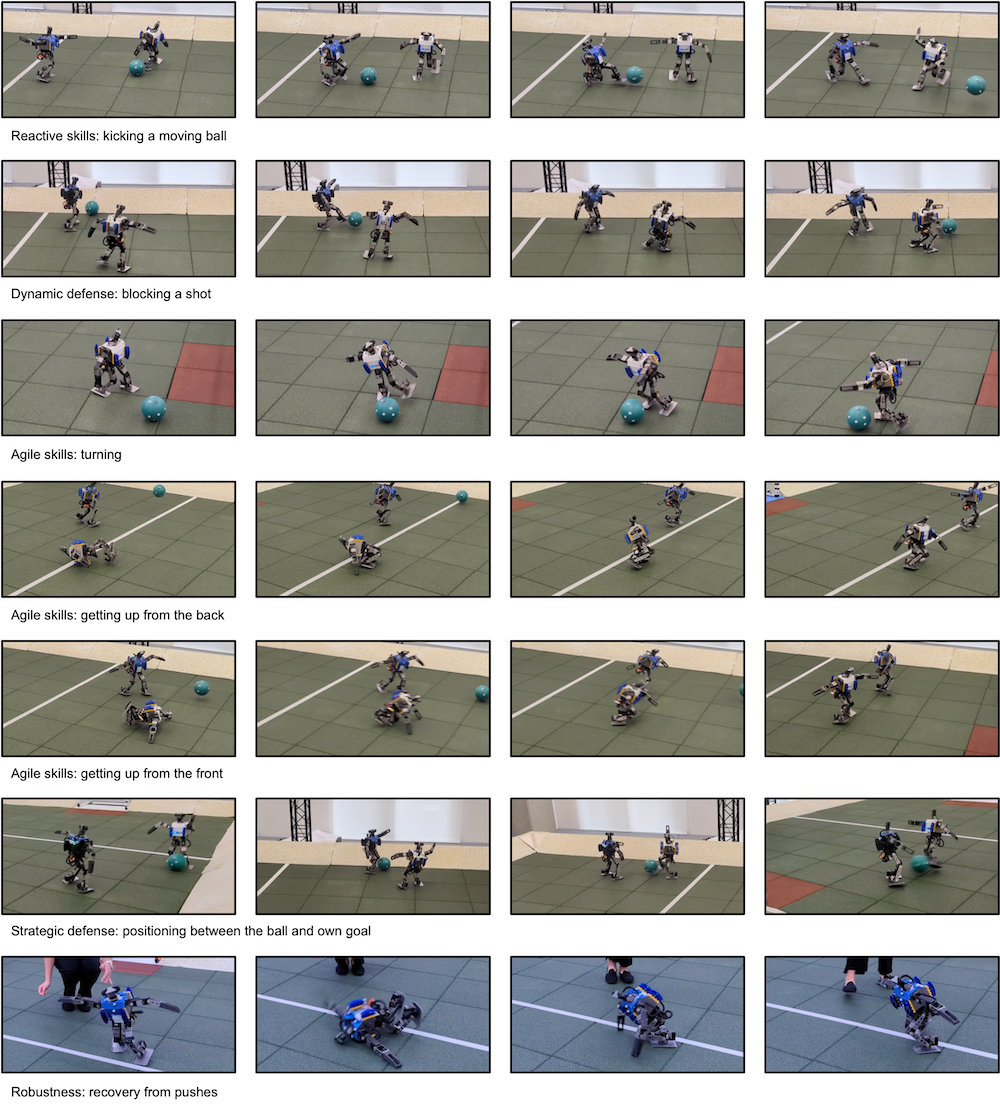
There’s nonetheless some work to do earlier than DeepMind-powered robots make it to the RoboCup. For these preliminary exams, researchers fully relied on simulation-based deep RL coaching earlier than transferring that info to bodily robots. Sooner or later, engineers wish to mix each digital and real-time reinforcement coaching for his or her bots. In addition they hope to scale up their robots, however that can require way more experimentation and fine-tuning.
The crew believes that using comparable deep RL approaches for soccer, in addition to many different duties, might additional enhance bipedal robots actions and real-time adaptation capabilities. Nonetheless, it’s unlikely you’ll want to fret about DeepMind humanoid robots on full-sized soccer fields—or within the labor market—simply but. On the identical time, given their steady enhancements, it’s most likely not a nasty thought to get able to blow the whistle on them.